Cognitive Biases in Large Language Models
David Thorstad, 2024-25 RPW Center Faculty Fellow. This year’s group is exploring the theme of Emerging Technologies in Human Context: Past, Present, and Future
It is often held that humans fall prey to a number of cognitive biases. For example, we may react differently to different framings of the same decision problem, or may anchor too strongly on one piece of information at the expense of others.
Recent work in computer science has argued that large language models may also display similar cognitive biases. In particular, many large language models appear to show humanlike cognitive biases in the same experimental paradigms used to measure human bias.
For example, in a classic experiment, Amos Tversky and Daniel Kahneman (1981) asked experiments to consider one of two versions of what appears to be the same scenario. In both cases, they were told that a disease looks likely to kill 600 people (`Common instructions’) and presented with two solutions, Program A and Program B. One group was told how many people would be saved by each solution (`Positive frame’) and the second group was told how many people would die in each solution (`Negative frame’).
Kahneman and Tversky found a framing effect: people favored different programs depending on which frame they were given. That is surprising, since the programs have not changed, so it looks like an irrational bias to change which program is favored based on how the program is framed.
More surprisingly, Alaina Talboy and Elizabeth Fuller (2023) found the same pattern in the outputs of large language models. This suggests that large language models may show humanlike cognitive biases, and similar studies have argued that other common biases may replicate in leading models.
This development is exciting because it provides a new way to assess the performance of large language models. Traditional studies of algorithmic bias use a notion of bias closely tied to unfairness, especially as affecting marginalized groups. Algorithms often perform badly on this metric, and this should not be ignored or downplayed. However, it may be that existing models perform better when we turn our sights to cognitive bias. In this paper, I provide some reason to think that they do.
My approach is rooted in the framework of bounded rationality. Bounded rationality theorists propose two reactions to allegations of cognitive bias in human cognition. The first and most prominent reaction is optimism. Optimists claim that many allegations of cognitive bias should be attributed to a `bias bias’ within the study of judgment and decisionmaking, rather than genuine bias within human agents. Optimists use a range of strategies to argue that claimed biases are non-existent, ill-formed, or in fact desirable when viewed in the proper light.
A second reaction to allegations of cognitive bias is anti-Panglossian meliorism. Theories of bounded rationality are frequently accused of siding with the good Doctor Pangloss in thinking that we live in the best of all possible worlds, in the sense that humans exhibit no cognitive biases whatsoever. But bounded rationalist theorists are no followers of Pangloss. Bounded rationality theorists avoid the charge of Panglossianism through an anti-Panglossian willingness to accept some well-evidenced biases as empirically genuine and normatively problematic.
My paper, “Cognitive bias in large language models: Cautious optimism meets anti-Panglossian meliorism,” extends both of these reactions from human cognition to the emerging study of cognitive bias within large language models.
Witnessing optimism, I consider three case studies of biases that have recently been alleged: knowledge effects in conditional reasoning by text generation models, availability bias in relation extraction from drug-drug interaction data, and anchoring bias in code generation. I propose strategies for rationalizing each of the claimed biases.
Witnessing anti-Panglossian meliorism, I return to the Talboy and Fuller (2023) study of framing effects from the beginning of this post. I argue that there is good evidence for the existence of framing effects in this case. I also argue that these framing effects are undesirable.
I conclude by mapping directions for future research in the study of cognitive bias in large language models, as well as by comparing current findings on cognitive bias to the existing literature on algorithmic bias.
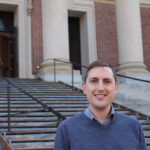
David Thorstad is Assistant Professor of Philosophy at Vanderbilt University, Senior Research Affiliate at the Global Priorities Institute, Oxford, and Research Affiliate at the MINT Lab, ANU. Thorstad’s research deals with bounded rationality, global priorities research, and the ethics of emerging technologies. His research asks how bounded agents should figure out what to do and believe, and how the answers to those questions bear on the allocation of philanthropic resources and the ethics of emerging technologies. Thorstad is the author of Inquiry under Bounds (Oxford, forthcoming) and co-editor of Essays on Longtermism (Oxford, forthcoming).